Creating AI Tutors That Don’t Hallucinate
Cracking the AI hallucination problem is all about the right training data and human input.
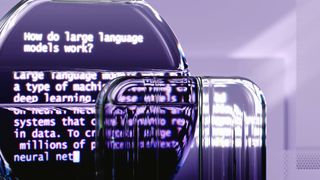
One of Google’s AI tools recently advised a user to make pizza with glue, Microsoft’s Bing chatbot famously told a New York Times writer he was unhappy in his marriage, and those who use other AI platforms regularly are likely to encounter similar inaccuracies. I recently asked GPT-4o for a realistic painting of New York City: It created an image with multiple Empire State Buildings.
These common AI hallucinations are exactly what professors Michael Klymkowsky and Ann Riedel are hoping to avoid when they use AI for teaching.
Klymkowsky, a professor at the University of Colorado Boulder, and Riedel, a professor at Front Range Community College in Colorado, are running a pilot study of two AI tools. The first is “Rita,” a chatbot tutor designed to engage in Socratic dialog with introductory biology students to increase inclusivity. The second tool is Rita’s analysis bot, “Dewey,” which is designed to assist instructors in assessing student work, not for grading but so that the teacher can identify student misconceptions and questions, and adjust their instruction accordingly.
“It’s really two functions,” Klymkowsky says of the tools. “One to help the instructor and liberate them from the idea that they have to fill everything up without knowing whether students really get it. And it's a help for the student to have a Socratic responder who will make them think about what they're talking about.”
However, for either of these chatbots to work as designed, avoiding hallucinations is key. Here’s how Klymkowsky and Riedel are working to overcome AI hallucinations.
AI Tutors That Don’t Hallucinate: Accurate Inputs
One of the reasons AI models hallucinate is because these are trained on vast amounts of internet data — and as anyone who spent time on social media or Reddit knows, the internet is not always a reliable narrator about, well, anything.
To overcome this, Rita is trained exclusively on vetted and accurate content. “It's constrained by the materials we're using,” Klymkowsky says. These include biology textbooks and peer-reviewed papers that Klymkowsky co-authored.
Tech & Learning Newsletter
Tools and ideas to transform education. Sign up below.
To train an AI chatbot on this more limited and specific data set, Klymkowsky is working with CustomGPT.ai. Klymkowsky and Riedel were awarded a grant from the technology company for their pilot AI tutor program that gives them premium access to the tool and other support.
CustomGPT.ai utilizes GPT-4 technology but is dedicated to eliminating AI hallucinations for its users. Alden Do Rosario, the company’s CEO and founder, says that previously in tech there was a saying that companies need to be security first or privacy first; however, he says AI platforms "need to be anti-hallucination first.”
Human Input
Allowing for users to train AI models on specific data is the first major step toward preventing AI hallucinations, Rosario says, but there’s another equally important factor that can too often be overlooked in tech design: human input.
“Unlike most software [problems], hallucination is one of those things where human element is required,” Rosario says. “You cannot just put an engineer in a dark room and tell him, ‘Hey, go solve hallucinations.’”
Instead, human experts need to help inform engineers of when AI tools are hallucinating. Rosario says when his platform users let the development team know about hallucinations, they study the root cause of the hallucination and improve the AI tool to help prevent that type of mistake from happening across all users. He likens this process to when a car company learns about a defect and issues a recall to owners.
Rosario adds it's a part of the development process that isn’t happening as much as it should be with AI. “It is quite ignored because even the big companies like OpenAI think, ‘We’ll throw an engineer at it, and they'll figure it out,'" he says.
Beyond Accuracy
So far these two factors have resulted in chatbots that Klymkowsky is comfortable deploying with students and teachers. While both his chatbots are still being studied and tested, Klymkowsky hasn’t come across any hallucinations. However, he acknowledges that creating an AI science chatbot might be less challenging than in some other academic fields where debate and nuance are more common.
“It's easier in the sciences because there are things that we know, and they're not ambiguous,” he says.
In addition, there are challenges beyond accuracy to creating effective AI tutors. For instance, the Rita chatbot is designed to engage students in conversation rather than just give them answers. “If the student is making an incorrect assumption or leaving something out, it’s saying, ‘Have you thought about this?’ or ‘Does this idea change the way you would answer?’” Klymkowsky says.
How to make sure these conversations are natural and engaging to students is an ongoing process, Klymkowsky says. “You don't want the bot to lecture at them,” he says. “In fact, that's probably the biggest challenge, stopping the bot from lecturing to them.”
Solving that problem is important but less vital as most educators can live with students using a boring tutor. It's an inaccurate tutor we worry about.
Erik Ofgang is a Tech & Learning contributor. A journalist, author and educator, his work has appeared in The New York Times, the Washington Post, the Smithsonian, The Atlantic, and Associated Press. He currently teaches at Western Connecticut State University’s MFA program. While a staff writer at Connecticut Magazine he won a Society of Professional Journalism Award for his education reporting. He is interested in how humans learn and how technology can make that more effective.